Techniques like red teaming, where expert testers try to elicit unsafe behaviors, can surface vulnera- bilities. Careful prompt engineering can also help assess models’ resilience against malicious prompts or out-of-distribution inputs. The results highlight the importance of continuous monitoring, as models can degrade or exhibit new issues over time. Over 40% of respondents evaluate their models following any changes or prior to major releases, highlighting the shift towards a continuous evaluation that goes beyond one-time assessments. While model evaluation plays a crucial role in measuring AI performance, leaders responsible for applying AI in their organizations must also demon- strate tangible business outcomes. Almost half of respondents evaluate models based on their direct impact on KPIs like operational efficiency or customer satisfaction. Grounding evaluations in downstream outcomes ensures that models are not just technically proficient but actually valuable in practice. 40 41
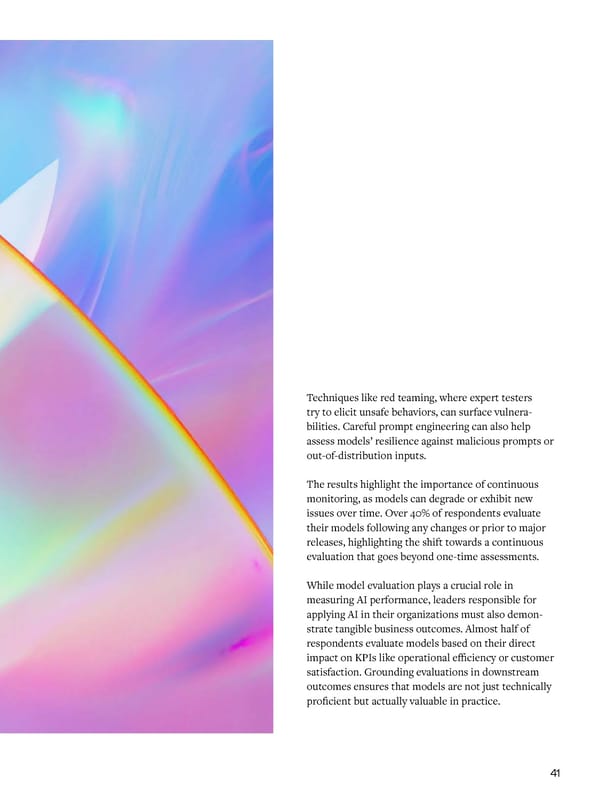