AI Readiness Report 2024
The report titled 'Zeitgeist' explores the state of AI readiness and strategies for the upcoming year.
AI READINESS REPORT 2024 1
Introduction The hype for generative AI has reached its peak. Developers continue to push the limits, exploring new frontiers with Table of increasingly sophisticated models. At the same time, without a standardized blueprint, enterprises and governments are Contents grappling with the risks vs. rewards that come with adopting AI. That’s why in our third edition of Scale Zeitgeist: lacking security benchmarks, and 50% desired AI Readiness Report, we focused on what it takes industry-specific benchmarks. Additionally, while AI Year in Review 4 to transition from merely adopting AI to actively 79% of respondents cited improving operational optimizing and evaluating it. To understand the efficiency as the key reason for adopting AI, only Apply AI 13 state of AI development and adoption today, half are measuring the business impact of their AI we surveyed more than 1,800 ML practitioners initiatives. And while performance and reliability Build AI 26 and leaders directly involved in building or (each at 69%) were indicated as the top reasons applying AI solutions and interviewed dozens for evaluating models, safety ranked lower (55%), more. In other words, we removed responses running counter to popular narratives. Evaluate AI 36 from business leaders or executives who are not equipped to know or understand the challenges This report presents expert insights from Scale Conclusion 46 of AI adoption first-hand. and its partners across the ecosystem, including frontier AI companies, enterprises, and govern- Methodology 47 Our findings show that of the 60% of respon- ments. Whether you are developing your own dents who have not yet adopted AI, security models (building AI), leveraging existing foun- concerns and lack of expertise were the top two dation models (applying AI), or testing models reasons holding them back. This finding seems to (evaluating AI), there are actionable insights and validate the “AI safety” narrative that dominates best practices for everyone. today’s news. Among survey respondents who have adopted AI, many feel they lack the appro- priate benchmarks to effectively evaluate models. Specifically, 48% of respondents referenced ii 01
Introduction The hype for generative AI has reached its peak. Developers continue to push the limits, exploring new frontiers with Table of increasingly sophisticated models. At the same time, without a standardized blueprint, enterprises and governments are Contents grappling with the risks vs. rewards that come with adopting AI. That’s why in our third edition of Scale Zeitgeist: lacking security benchmarks, and 50% desired AI Readiness Report, we focused on what it takes industry-specific benchmarks. Additionally, while AI Year in Review4to transition from merely adopting AI to actively 79% of respondents cited improving operational optimizing and evaluating it. To understand the efficiency as the key reason for adopting AI, only Apply AI13state of AI development and adoption today, half are measuring the business impact of their AI we surveyed more than 1,800 ML practitioners initiatives. And while performance and reliability Build AI 26and leaders directly involved in building or (each at 69%) were indicated as the top reasons applying AI solutions and interviewed dozens for evaluating models, safety ranked lower (55%), more. In other words, we removed responses running counter to popular narratives. Evaluate AI 36from business leaders or executives who are not equipped to know or understand the challenges This report presents expert insights from Scale Conclusion46of AI adoption first-hand. and its partners across the ecosystem, including frontier AI companies, enterprises, and govern- Methodology47Our findings show that of the 60% of respon- ments. Whether you are developing your own dents who have not yet adopted AI, security models (building AI), leveraging existing foun- concerns and lack of expertise were the top two dation models (applying AI), or testing models reasons holding them back. This finding seems to (evaluating AI), there are actionable insights and validate the “AI safety” narrative that dominates best practices for everyone. today’s news. Among survey respondents who have adopted AI, many feel they lack the appro- priate benchmarks to effectively evaluate models. Specifically, 48% of respondents referenced ii 01
“The rapid evolution of AI offers both immense opportunities and challenges. Embracing it responsibly, with robust infrastructure and rigorous evaluation protocols, unlocks the potential of AI while safeguarding against the risks, known and unknown.” Alexandr Wang, FOUNDER & CEO, SCALE 02 03
“The rapid evolution of AI offers both immense opportunities and challenges. Embracing it responsibly, with robust infrastructure and rigorous evaluation protocols, unlocks the potential of AI while safeguarding against the risks, known and unknown.” Alexandr Wang, FOUNDER & CEO, SCALE 02 03
Genertive AI continues to reshpe our world Advancements in generative AI continued to accelerate in 2023. After the release of OpenAI’s ChatGPT in November 2022, the platform reached an estimated 100 million users in just two months. In March 2023, OpenAI released GPT-4, a large language multimodal model that demon- strated human-level performance across industry benchmarks. Other model builders joined the launch party last year. Google launched Bard, initially running on the LaMDA model and replaced shortly after by PaLM 2 (with improved domain-specific knowledge - including coding Yer in and math). Anthropic introduced Claude 2 in the summer with a 100K context window. A week later, Meta unveiled Llama 2 and Code Llama, and included model weights and code for the pretrained model. Google DeepMind closed out 2023 with the release of Gemini - repre- senting a significant improvement in performance as the first model to outperform human experts on the Massive Multitask Language Under- Review standing (MMLU) test. Newer open source model families like Falcon, Mixtral, and DBRX demonstrated the possibility for local inference while innovating on model architecture to use far less compute. This year, in March 2024, Anthropic launched the family of Claude 3 models, doubling the context window. Just a few days later, Cohere released their Command R generative model - designed for scalability and long context tasks. Frontier research underlies many of these model advancements. Some significant advancements include: 1. Open AI achieved improvements in mathematical reasoning through rewarding chain-of-thought reasoning. Scale contributed to the creation of PRM800K, the full process supervision dataset released as part of this paper. 2. Anthropic uncovered an approach for better model interpretabil- ity through analysis of feature activation compared to individual neurons. 3. The Microsoft Research team discovered that a model with a smaller number of parameters relative to state-of-the-art models can demon- strate impressive performance on task-specific benchmarks when fine-tuned with high-quality textbook data. 04 05
Genertive AI continues to reshpe our world Advancements in generative AI continued to accelerate in 2023. After the release of OpenAI’s ChatGPT in November 2022, the platform reached an estimated 100 million users in just two months. In March 2023, OpenAI released GPT-4, a large language multimodal model that demon- strated human-level performance across industry benchmarks. Other model builders joined the launch party last year. Google launched Bard, initially running on the LaMDA model and replaced shortly after by PaLM 2 (with improved domain-specific knowledge - including coding Yer in and math). Anthropic introduced Claude 2 in the summer with a 100K context window. A week later, Meta unveiled Llama 2 and Code Llama, and included model weights and code for the pretrained model. Google DeepMind closed out 2023 with the release of Gemini - repre- senting a significant improvement in performance as the first model to outperform human experts on the Massive Multitask Language Under- Review standing (MMLU) test. Newer open source model families like Falcon, Mixtral, and DBRX demonstrated the possibility for local inference while innovating on model architecture to use far less compute. This year, in March 2024, Anthropic launched the family of Claude 3 models, doubling the context window. Just a few days later, Cohere released their Command R generative model - designed for scalability and long context tasks. Frontier research underlies many of these model advancements. Some significant advancements include: 1. Open AI achieved improvements in mathematical reasoning through rewarding chain-of-thought reasoning. Scale contributed to the creation of PRM800K, the full process supervision dataset released as part of this paper. 2. Anthropic uncovered an approach for better model interpretabil- ity through analysis of feature activation compared to individual neurons. 3. The Microsoft Research team discovered that a model with a smaller number of parameters relative to state-of-the-art models can demon- strate impressive performance on task-specific benchmarks when fine-tuned with high-quality textbook data. 04 05
Key findings, 2023 to 2024 To illustrate the evolving landscape, we Do you customize generative AI models or use them out of the box? see the following changes as important Organizations reporting trends in AI over the past year. generative AI forced the creation of an AI strategy: Organizations with no Organizations planning plans to work with to increase investment in generative AI: commercial and closed- source models over the next three years: Organizations that Organizations with consider AI to be very generative AI models in or highly critical to production: their business in the next three years: Organizations applying AI are seeking to extract specific tasks or datasets, significantly enhancing their additional value by optimizing AI through prompt-en- performance and accuracy on targeted applications. 2023 2024 gineering, fine-tuning models, and retrieval augmented RAG further enhances this by dynamically incorporat- 2024 generation (RAG). Despite the desire to optimize foun- ing external information during the generation process, dational models, 65% of organizations use models out- enabling the model to produce more relevant and con- of-the-box, 43% of organizations fine-tune models and textually rich outputs. 38% use RAG. Fine-tuning can customize models for 06 07
Key findings, 2023 to 2024 To illustrate the evolving landscape, we Do you customize generative AI models or use them out of the box? see the following changes as important Organizations reporting trends in AI over the past year.generative AI forced the creation of an AI strategy: Organizations with no Organizations planning plans to work with to increase investment in generative AI:commercial and closed- source models over the next three years: Organizations that Organizations with consider AI to be very generative AI models in or highly critical to production: their business in the next three years: Organizations applying AI are seeking to extract specific tasks or datasets, significantly enhancing their additional value by optimizing AI through prompt-en- performance and accuracy on targeted applications. 20232024 gineering, fine-tuning models, and retrieval augmented RAG further enhances this by dynamically incorporat- 2024 generation (RAG). Despite the desire to optimize foun- ing external information during the generation process, dational models, 65% of organizations use models out- enabling the model to produce more relevant and con- of-the-box, 43% of organizations fine-tune models and textually rich outputs. 38% use RAG. Fine-tuning can customize models for 06 07
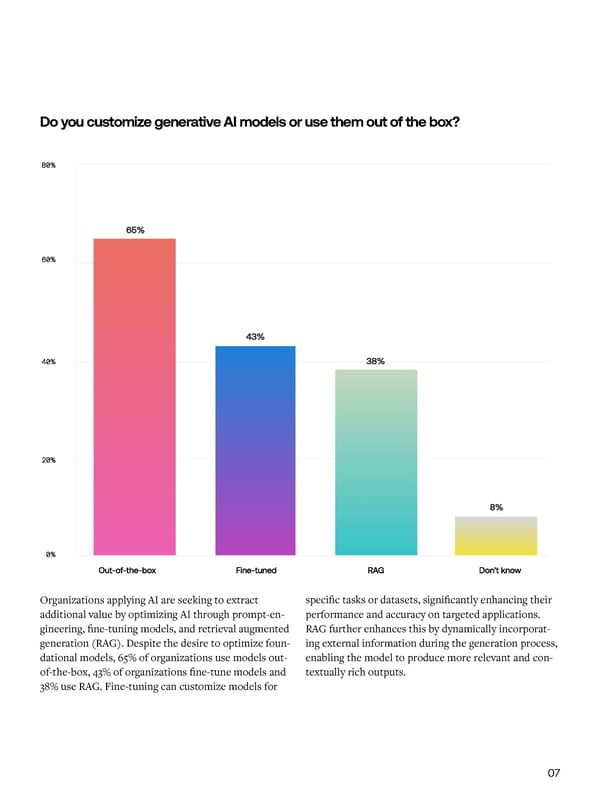
How do you work with generative AI models? What positive outcomes have you seen from generative AI adoption? Model preferences continue to usage of closed-source models Similar to last year, 61% of orga- Processes like RAG and fine-tuning Proprietary data is a key ingredient evolve and remain a key decision easier. Many closed-source models nizations stated improved oper- introduce the complexity of integrat- to power performance enhance- for an organization’s AI strategy. also outperform open source ational efficiency as the leading ing external data sources in real- ments for generative AI models. The largest increase in usage came models out-of-the-box. Despite driver behind adopting generative time, ensuring the relevance and While Scale’s machine learning from closed-source models with that, open-source model usage still AI. Improved customer experience accuracy of retrieved information, team proved how fine-tuning can 86% of organizations using these increased from 41% to 66%. This is came in second at 55%. managing additional computation- enhance model capabilities, 41% of models compared to 37% the year likely due to the flexibility open- al costs, and addressing potential organizations lack the ML expertise prior. This is likely due to a combi- source models provide for fine-tun- Despite growing adoption, there are biases or errors. Fine-tuning requires to execute the data transformations nation of factors. Many organiza- ing and hosting. The smallest still a number of challenges that stall careful selection of data to avoid and measure and evaluate results to tions have existing contracts with change in model preferences were widespread use of generative AI. 61% overfitting and ensuring models justify the initial investment. cloud service providers who in turn organizations that trained their of respondents cited infrastructure, remain generalizable to new, unseen have partnerships with closed- own models at 24% in 2024. tooling, or out-of-the-box solutions information. source model developers, making not meeting their specific needs. 08 09
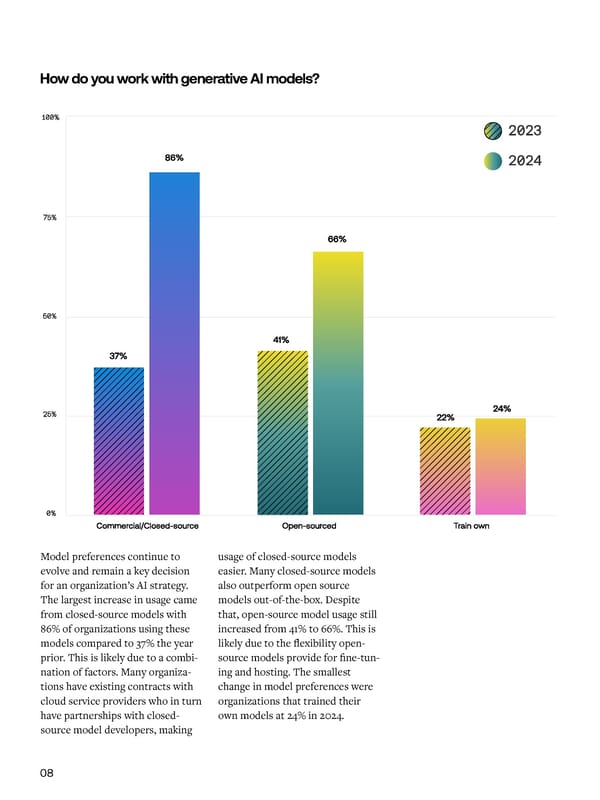
How do you work with generative AI models?What positive outcomes have you seen from generative AI adoption? Model preferences continue to usage of closed-source models Similar to last year, 61% of orga-Processes like RAG and fine-tuning Proprietary data is a key ingredient evolve and remain a key decision easier. Many closed-source models nizations stated improved oper-introduce the complexity of integrat-to power performance enhance- for an organization’s AI strategy. also outperform open source ational efficiency as the leading ing external data sources in real-ments for generative AI models. The largest increase in usage came models out-of-the-box. Despite driver behind adopting generative time, ensuring the relevance and While Scale’s machine learning from closed-source models with that, open-source model usage still AI. Improved customer experience accuracy of retrieved information, team proved how fine-tuning can 86% of organizations using these increased from 41% to 66%. This is came in second at 55%. managing additional computation-enhance model capabilities, 41% of models compared to 37% the year likely due to the flexibility open-al costs, and addressing potential organizations lack the ML expertise prior. This is likely due to a combi-source models provide for fine-tun-Despite growing adoption, there are biases or errors. Fine-tuning requires to execute the data transformations nation of factors. Many organiza-ing and hosting. The smallest still a number of challenges that stall careful selection of data to avoid and measure and evaluate results to tions have existing contracts with change in model preferences were widespread use of generative AI. 61% overfitting and ensuring models justify the initial investment. cloud service providers who in turn organizations that trained their of respondents cited infrastructure, remain generalizable to new, unseen have partnerships with closed-own models at 24% in 2024.tooling, or out-of-the-box solutions information. source model developers, making not meeting their specific needs. 08 09
Wht to Expect in 2024 Increasingly Capable Foundation Models Expert Insight Will Power Performance Evolving Proof-of-Concepts to Scaling Improvements Production Deployments In the coming year, we expect notable advancements As researchers continue to refine these models, we can in generative AI foundation models to continue. also anticipate improvements in accuracy and reduced Human experts will play an increasingly crucial role in Improvements in model performance and capabilities Models like Claude 3 have demonstrated improved latency, making models more reliable and efficient. model advancements and evaluation. As models start will motivate leaders to quickly iterate from proof-of- performance on various benchmarks, such as scoring The size of these foundation models is also likely to to exhaust the corpus of general information widely concepts to pilots to production deployments. More 86.8% on the MMLU dataset and 95.0% on the GSM8K grow, allowing them to capture and leverage even more available on the internet, models will require addi- user friendly RAG and fine-tuning solutions will emerge math problem set, indicating enhanced capabilities knowledge and nuance from the vast amounts of data tional data to improve their capabilities. While some as on-ramps to improve adoption so that organizations in reasoning and problem-solving. We also expect to they are trained on. organizations may look to replace human-generated can more easily customize models. As start up costs see the emergence of more sophisticated multimodal data with synthetic data for training, models reliant on taper, model effectiveness improves, and more robust models that can seamlessly integrate and generate synthetic data can be susceptible to model collapse. A evaluation strategies emerge, organizations will be able content across various modalities, including text, hybrid human and synthetic data approach can mitigate to more clearly capture and define return on invest- images, audio, and video as both inputs and outputs. biases from synthetic data and still reflect nuanced ment. human preferences. The domain-specific knowledge of experts allows them to provide data that captures the Increasing Emphasis on Test & Evaluation nuance, complexity, and diversity to supplement model Practices training. Experts are also critical for testing and eval- Evolution of generative AI capabilities: domain and functional capabilities are rapidly growing uation alongside reinforcement learning from human Nearly every major model release usurps a different Math Creative Coding Science feedback, with the knowledge to identify subtle errors, leading model on various benchmarks. Enterprises Writing inconsistencies, or biases in order to provide reliable will want to create their own evaluation methodology guidance to preferred model outputs. consisting of industry benchmarks, automated model metrics, and measures for return on investment to While experts are necessary to improve model capabili- continuously evaluate their preferred model. As model ties, we anticipate organizations defining new roles that capabilities grow, model builders will place more are centered around generative AI. Prompt engineers, importance on guardrails, steerability, safety, security, machine learning researchers, and generative AI and transparency. Public sector institutions now must experts will collaborate with subject matter experts to consider the White House’s OMB Policy and test and ensure AI initiatives are successful. Generative AI will evaluate AI systems to ensure that AI is safe. Multivariate Metaphorical Debugging Biology fundamentally change the nature of work. Calculus Stories Applying Lyrical Code Genetic Gradient Sonnets Optimization Expression Theorem 10 11
Wht to Expect in 2024 Increasingly Capable Foundation ModelsExpert Insight Will Power Performance Evolving Proof-of-Concepts to Scaling Improvements Production Deployments In the coming year, we expect notable advancements As researchers continue to refine these models, we can in generative AI foundation models to continue. also anticipate improvements in accuracy and reduced Human experts will play an increasingly crucial role in Improvements in model performance and capabilities Models like Claude 3 have demonstrated improved latency, making models more reliable and efficient. model advancements and evaluation. As models start will motivate leaders to quickly iterate from proof-of- performance on various benchmarks, such as scoring The size of these foundation models is also likely to to exhaust the corpus of general information widely concepts to pilots to production deployments. More 86.8% on the MMLU dataset and 95.0% on the GSM8K grow, allowing them to capture and leverage even more available on the internet, models will require addi-user friendly RAG and fine-tuning solutions will emerge math problem set, indicating enhanced capabilities knowledge and nuance from the vast amounts of data tional data to improve their capabilities. While some as on-ramps to improve adoption so that organizations in reasoning and problem-solving. We also expect to they are trained on. organizations may look to replace human-generated can more easily customize models. As start up costs see the emergence of more sophisticated multimodal data with synthetic data for training, models reliant on taper, model effectiveness improves, and more robust models that can seamlessly integrate and generate synthetic data can be susceptible to model collapse. A evaluation strategies emerge, organizations will be able content across various modalities, including text, hybrid human and synthetic data approach can mitigate to more clearly capture and define return on invest- images, audio, and video as both inputs and outputs. biases from synthetic data and still reflect nuanced ment. human preferences. The domain-specific knowledge of experts allows them to provide data that captures the Increasing Emphasis on Test & Evaluation nuance, complexity, and diversity to supplement model Practices training. Experts are also critical for testing and eval- Evolution of generative AI capabilities: domain and functional capabilities are rapidly growinguation alongside reinforcement learning from human Nearly every major model release usurps a different MathCreative CodingSciencefeedback, with the knowledge to identify subtle errors, leading model on various benchmarks. Enterprises Writing inconsistencies, or biases in order to provide reliable will want to create their own evaluation methodology guidance to preferred model outputs. consisting of industry benchmarks, automated model metrics, and measures for return on investment to While experts are necessary to improve model capabili- continuously evaluate their preferred model. As model ties, we anticipate organizations defining new roles that capabilities grow, model builders will place more are centered around generative AI. Prompt engineers, importance on guardrails, steerability, safety, security, machine learning researchers, and generative AI and transparency. Public sector institutions now must experts will collaborate with subject matter experts to consider the White House’s OMB Policy and test and ensure AI initiatives are successful. Generative AI will evaluate AI systems to ensure that AI is safe. Multivariate Metaphorical DebuggingBiologyfundamentally change the nature of work. CalculusStories Applying Lyrical Code Genetic Gradient SonnetsOptimizationExpression Theorem 10 11
Apply AI Adoption Trends In a world where innovation moves at the speed of thought, generative AI has emerged as a transformative force. En- terprises and governments are deploying resources, capital, and teams to not just embed models into business processes, but also transform the paradigm of industry operations. This section highlights trends in enter- prise AI, including stages of adoption, model preferences, and investment themes for model categories. We’ll also dig into leading enterprise AI use-cases, the challenges behind AI adoption, and uncover the barriers that prevent organi- zations from using AI. 1212 1313
Apply AI Adoption Trends In a world where innovation moves at the speed of thought, generative AI has emerged as a transformative force. En- terprises and governments are deploying resources, capital, and teams to not just embed models into business processes, but also transform the paradigm of industry operations. This section highlights trends in enter- prise AI, including stages of adoption, model preferences, and investment themes for model categories. We’ll also dig into leading enterprise AI use-cases, the challenges behind AI adoption, and uncover the barriers that prevent organi- zations from using AI. 1212 1313
What is the current stage of your AI/ML project? Which of the following describes how your company works with generative AI models? No model deployed to production 25% 4% Evaluating use cases No plans to work with generative AI models One or more models deployed 38% 26% Generative AI models Developing the first in production model/application 22% 25% One model/ Plan on working with application deployed generative AI models to production 27% 33% Multiple models/ Experimented with applications deployed generative AI models to production The Evolution of AI Adoption 22% of organizations have one businesses can adapt to evolving Many organizations are increasingly Application and model develop- Technical organizations are model in production with 27% requirements while mitigating risks dedicating time to evaluating use ment follows use case selection. ahead of the curve with genera- of total respondents reporting associated with relying on a single cases to ensure alignment with Deploying generative AI in an tive AI adoption. Software and multiple models in production. model. The growing number of business objectives. Thorough use enterprise setting involves a internet companies are leading the Deploying multiple generative AI models in production reflects the case evaluation allows companies multi-step process, including data pack with 48% of organizations models in production allows orga- progression of proof-of-concepts to to identify applications with high preparation and pre-processing, reporting generative AI models in nizations to leverage specialized production deployments. ROI potential, assess feasibility and model selection and architecture production. Conversely, only 24% capabilities, avoid vendor lock-in, risk, and prioritize implementation design, hyperparameter tuning of government and defense entities and scale multiple use-cases. By 49% of organizations are still either efforts. and training, API development for have generative AI models in pro- comparing performance across evaluating use cases or develop- integration, monitoring feedback, duction. models and maintaining flexibility, ing the first model or application. and test and evaluation. 14 15
What is the current stage of your AI/ML project?Which of the following describes how your company works with generative AI models? No model deployed to production 25% 4% Evaluating use cases No plans to work with generative AI models One or more models deployed 38% 26% Generative AI models Developing the first in production model/application22%25% One model/ Plan on working with application deployed generative AI models to production 27% 33% Multiple models/ Experimented with applications deployed generative AI models to production The Evolution of AI Adoption 22% of organizations have one businesses can adapt to evolving Many organizations are increasingly Application and model develop-Technical organizations are model in production with 27% requirements while mitigating risks dedicating time to evaluating use ment follows use case selection. ahead of the curve with genera- of total respondents reporting associated with relying on a single cases to ensure alignment with Deploying generative AI in an tive AI adoption. Software and multiple models in production. model. The growing number of business objectives. Thorough use enterprise setting involves a internet companies are leading the Deploying multiple generative AI models in production reflects the case evaluation allows companies multi-step process, including data pack with 48% of organizations models in production allows orga-progression of proof-of-concepts to to identify applications with high preparation and pre-processing, reporting generative AI models in nizations to leverage specialized production deployments. ROI potential, assess feasibility and model selection and architecture production. Conversely, only 24% capabilities, avoid vendor lock-in, risk, and prioritize implementation design, hyperparameter tuning of government and defense entities and scale multiple use-cases. By 49% of organizations are still either efforts. and training, API development for have generative AI models in pro- comparing performance across evaluating use cases or develop-integration, monitoring feedback, duction. models and maintaining flexibility, ing the first model or application. and test and evaluation. 14 15
Which generative AI models do you work with? Note - at the time of the survey, Claude 3, Grok, and DallE3 were not released and thus not included in the survey. Model Preferences Model selection is critical for generative AI devel- infrastructure, managed services, and per token inputs Our respondents indicate that their preferred model is opment, as it determines the system’s performance, and outputs. OpenAI is overwhelmingly the preferred the latest version of OpenAI GPT- 4 with 58% of enter- scalability, and alignment with specific task require- model vendor. Virality and the ongoing rollout of prises using the latest version and 44% of enterprises ments, data characteristics, computational resources, advanced features positioned OpenAI as the preferred using GPT-3.5. Trailing closely behind, 39% of enterprises and trade-offs between model complexity and inference model vendor even as other models demonstrate com- use Google Gemini. There’s a notable drop-off in model speed. Organizations also evaluate model selection parable performance. selection following these three models with OpenAI through cost trade-offs - comparing investments tied to GPT-3 at 26%. 16 17
Which generative AI models do you work with? Note - at the time of the survey, Claude 3, Grok, and DallE3 were not released and thus not included in the survey. Model Preferences Model selection is critical for generative AI devel-infrastructure, managed services, and per token inputs Our respondents indicate that their preferred model is opment, as it determines the system’s performance, and outputs. OpenAI is overwhelmingly the preferred the latest version of OpenAI GPT- 4 with 58% of enter- scalability, and alignment with specific task require-model vendor. Virality and the ongoing rollout of prises using the latest version and 44% of enterprises ments, data characteristics, computational resources, advanced features positioned OpenAI as the preferred using GPT-3.5. Trailing closely behind, 39% of enterprises and trade-offs between model complexity and inference model vendor even as other models demonstrate com-use Google Gemini. There’s a notable drop-off in model speed. Organizations also evaluate model selection parable performance.selection following these three models with OpenAI through cost trade-offs - comparing investments tied to GPT-3 at 26%. 16 17
How does your company plan on investing in generative AI over the next 3 years? In which ways has your company implemented AI? Model Investment Deploying nd Customizing AI Use Cses Just as the leading preferred models leading commercial closed-source improved operational efficiency Coding copilots are becoming Organizations can optimize genera- (RAG) - enhancing the model’s are closed-source commercial models are closely tied to leading is the key driver behind adopting mainstream with technical users tive AI models for specific use cases knowledge by integrating infor- models, planned investments in cloud-service providers. Enter- generative AI. Generative AI use being early adopters of solutions through the following techniques: mation from external sources these categories of models reflect prises can draw down from cloud cases reflect this shift in priorities. like GitHub Copilot, CodeLlama, during the generation process. usage trends. 72% of organizations spend commitments through use of The leading use-cases for generative and Devin. Model vendors have • Prompt-engineering - guiding plan to increase investments in partner models (e.g., Amazon and AI adoption are computer program- responded to demand for content the model’s output through Teams are likely to maximize their commercial closed-source models. Anthropic, Microsoft and Open AI). ming and content generation.. generation with prompt templates carefully crafted input prompts AI investments by adopting these A lower percentage of organiza- that guide users to effective content techniques. For organizations that tions plan to invest in open-source Last year, organizations referenced creation questions for functions • Fine-tuning - training the model already fine-tune their own models, models at 67%. While open- the ability to develop new products including Marketing, Product Man- on domain-specific data 39% saw improved performance on source models provide organiza- or services as the leading reason agement, and Public Relations. domain-specific tasks compared to tions with greater control, many to adopt generative AI. This year, • Retrieval-Augmented Generation out-of-the-box models. 18 19
How does your company plan on investing in generative AI over the next 3 years?In which ways has your company implemented AI? Model Investment Deploying nd Customizing AI Use Cses Just as the leading preferred models leading commercial closed-source improved operational efficiency Coding copilots are becoming Organizations can optimize genera-(RAG) - enhancing the model’s are closed-source commercial models are closely tied to leading is the key driver behind adopting mainstream with technical users tive AI models for specific use cases knowledge by integrating infor- models, planned investments in cloud-service providers. Enter-generative AI. Generative AI use being early adopters of solutions through the following techniques:mation from external sources these categories of models reflect prises can draw down from cloud cases reflect this shift in priorities. like GitHub Copilot, CodeLlama, during the generation process. usage trends. 72% of organizations spend commitments through use of The leading use-cases for generative and Devin. Model vendors have • Prompt-engineering - guiding plan to increase investments in partner models (e.g., Amazon and AI adoption are computer program-responded to demand for content the model’s output through Teams are likely to maximize their commercial closed-source models. Anthropic, Microsoft and Open AI). ming and content generation..generation with prompt templates carefully crafted input promptsAI investments by adopting these A lower percentage of organiza-that guide users to effective content techniques. For organizations that tions plan to invest in open-source Last year, organizations referenced creation questions for functions • Fine-tuning - training the model already fine-tune their own models, models at 67%. While open-the ability to develop new products including Marketing, Product Man-on domain-specific data39% saw improved performance on source models provide organiza-or services as the leading reason agement, and Public Relations.domain-specific tasks compared to tions with greater control, many to adopt generative AI. This year, • Retrieval-Augmented Generation out-of-the-box models. 18 19
“With fine-tuning, there’s always the issue of data that we fine-tune on and compute. We can address hallucination and bias with better data. Frequency of fine-tuning helps but it’s an expensive procedure, most of the work that happens is on the data-side. We’re always on the search for more volume of data and better annotations.” Mohammed Minhaas, DATA ENGINEER 20 21
“With fine-tuning, there’s always the issue of data that we fine-tune on and compute. We can address hallucination and bias with better data. Frequency of fine-tuning helps but it’s an expensive procedure, most of the work that happens is on the data-side. We’re always on the search for more volume of data and better annotations.” Mohammed Minhaas, DATA ENGINEER 20 21
What are the top challenges in implementing AI technologies at your company? If you have not yet adopted AI, why have you not adopted it? Brriers to AI Adoption nd Implementtion Despite rapid advancements in the systems, can hinder the scalability use vast amounts of potentially field, organizations still face challeng- and efficiency of AI implementations, sensitive training data. The risk of es with AI implementation. 61% of leading to increased complexity and data breaches, unauthorized access, organizations specified that infra- higher costs. or misuse of personal information structure, tooling, or out-of-the-box during the data collection, storage, solutions don’t meet their needs. 54% of organizations struggle with and processing stages can expose Insufficient tooling for tasks such insufficient budget. Finding a home organizations to legal liabilities and as data preparation, model training, on the balance sheet for new gener- reputational damage, particularly in and deployment, combined with the ative AI projects limits the pace of industries with stringent data protec- lack of standardized frameworks for adoption. 52% also have concerns tion regulations. For example, certain integrating generative AI into existing about data privacy. Fine-tuning can health and human service providers 22 23
What are the top challenges in implementing AI technologies at your company?If you have not yet adopted AI, why have you not adopted it? Brriers to AI Adoption nd Implementtion Despite rapid advancements in the systems, can hinder the scalability use vast amounts of potentially field, organizations still face challeng- and efficiency of AI implementations, sensitive training data. The risk of es with AI implementation. 61% of leading to increased complexity and data breaches, unauthorized access, organizations specified that infra- higher costs. or misuse of personal information structure, tooling, or out-of-the-box during the data collection, storage, solutions don’t meet their needs. 54% of organizations struggle with and processing stages can expose Insufficient tooling for tasks such insufficient budget. Finding a home organizations to legal liabilities and as data preparation, model training, on the balance sheet for new gener- reputational damage, particularly in and deployment, combined with the ative AI projects limits the pace of industries with stringent data protec- lack of standardized frameworks for adoption. 52% also have concerns tion regulations. For example, certain integrating generative AI into existing about data privacy. Fine-tuning can health and human service providers 22 23
“RAG aims to address a key challenge with LLMs - while they are very creative, they lack factual understanding of the world and struggle to explain their reasoning. RAG tackles this by connecting LLMs to known data sources, like a bank’s general ledger, using vector search on a database. This augments the LLM prompts with relevant facts. However, implementing RAG presents its own challenges. It requires creating and maintaining the external data connection, setting up a fast vector database, and designing vector representations of the data for efficient search. Companies need to consider if they require a purpose-built database optimized for vector search. Keeping this vectorized representation of truth up-to-date is tricky. As the underlying data sources change over time and users ask new questions, the vector database needs to evolve as well. Deciding if and how to incorporate user assumptions into the vector representations is a philosophical question that also has practical implications for implementation. The industry is still grappling with how to design RAG systems that can continually improve over time.” Jon Barker, CUSTOMER ENGINEER, GOOGLE 24 25
“RAG aims to address a key challenge with LLMs - while they are very creative, they lack factual understanding of the world and struggle to explain their reasoning. RAG tackles this by connecting LLMs to known data sources, like a bank’s general ledger, using vector search on a database. This augments the LLM prompts with relevant facts. However, implementing RAG presents its own challenges. It requires creating and maintaining the external data connection, setting up a fast vector database, and designing vector representations of the data for efficient search. Companies need to consider if they require a purpose-built database optimized for vector search. Keeping this vectorized representation of truth up-to-date is tricky. As the underlying data sources change over time and users ask new questions, the vector database needs to evolve as well. Deciding if and how to incorporate user assumptions into the vector representations is a philosophical question that also has practical implications for implementation. The industry is still grappling with how to design RAG systems that can continually improve over time.” Jon Barker, CUSTOMER ENGINEER, GOOGLE 24 25
Build AI Pushing the Boundries: AI’s Rpid Advncement Across Domins As highlighted in the Year In Review section of this report, we’ve seen a significant leap in model capabilities in the past year. The latest models have revolutionized programming, writing clean, efficient code from natural language prompts with an almost human-like understanding of intent. But the advancements don’t stop there. We’re not far away from a world where AI agents effortlessly communicate across language barriers, solve complex mathematical equations, explain scientific concepts, and even make new discoveries. Moreover, AI is rapidly advancing in its ability to perceive and generate content across multiple modalities, including text, images, audio, and video. 26 27
Build AI Pushing the Boundries: AI’s Rpid Advncement Across Domins As highlighted in the Year In Review section of this report, we’ve seen a significant leap in model capabilities in the past year. The latest models have revolutionized programming, writing clean, efficient code from natural language prompts with an almost human-like understanding of intent. But the advancements don’t stop there. We’re not far away from a world where AI agents effortlessly communicate across language barriers, solve complex mathematical equations, explain scientific concepts, and even make new discoveries. Moreover, AI is rapidly advancing in its ability to perceive and generate content across multiple modalities, including text, images, audio, and video. 26 27
The key pillars of The race between leaders like OpenAI, Anthropic, Google, Meta, and others is eective AI models driving the rapid advancement of foun- dation models. Each lab is pushing the boundaries of what’s possible, releasing new models that leapfrog the capabilities of predecessors. Developing industry-leading AI requires a combination of: However, the pace of releases is not constant. The survey data reveals that it typically takes companies three to six months to develop a model and deploy it to production. For the top labs, major releases are often spaced six to nine months apart, waiting until achieving a significant step-change in performance before unveiling a new model. We expect this six to nine month release cadence to continue over the coming year. However, the pace could decelerate as organizations encounter data limita- tions and struggle to achieve meaningful improvements over current models’ performance. The following sections will explore the key pillars needed to build effective models, including model architecture innovations, computational resource trends, and the high-quality data imper- ative. We’ll also discuss future invest- THOUGHTFUL VAST COMPUTATIONAL CAREFULLY ments and priorities in the AI landscape MODEL ARCHITECTURES RESOURCES CURATED DATASETS providing insights into the advance- ments shaping the future of AI. ChatGPT Galactica InstructGPT Bard Timeline of Model Releases Imagen Claude 1 DALL-E-2 LLaMA GPT-4 PaLM OPT-175B Claude 2 Meta Open AI Google Anthropic Codey Whisper Claude 3 Constitutional AI Minerva PaLM-SayCan Segment Anything BlenderBot DALL-E CODEX Med-PaLM PaLM 2 BERT GPT-2 RoBERTa GPT-3 CLIP Gemini Claude Instant 2019 2020 2021 2022 2023 2024 28 29
The key pillars of The race between leaders like OpenAI, Anthropic, Google, Meta, and others is eective AI models driving the rapid advancement of foun- dation models. Each lab is pushing the boundaries of what’s possible, releasing new models that leapfrog the capabilities of predecessors.Developing industry-leading AI requires a combination of: However, the pace of releases is not constant. The survey data reveals that it typically takes companies three to six months to develop a model and deploy it to production. For the top labs, major releases are often spaced six to nine months apart, waiting until achieving a significant step-change in performance before unveiling a new model. We expect this six to nine month release cadence to continue over the coming year. However, the pace could decelerate as organizations encounter data limita- tions and struggle to achieve meaningful improvements over current models’ performance. The following sections will explore the key pillars needed to build effective models, including model architecture innovations, computational resource trends, and the high-quality data imper- ative. We’ll also discuss future invest-THOUGHTFUL VAST COMPUTATIONAL CAREFULLY ments and priorities in the AI landscape MODEL ARCHITECTURES RESOURCES CURATED DATASETS providing insights into the advance- ments shaping the future of AI. ChatGPT Galactica InstructGPT Bard Timeline of Model Releases Imagen Claude 1 DALL-E-2 LLaMA GPT-4 PaLM OPT-175B Claude 2 MetaOpen AIGoogleAnthropic Codey Whisper Claude 3 Constitutional AI Minerva PaLM-SayCan Segment Anything BlenderBotDALL-ECODEX Med-PaLM PaLM 2 BERT GPT-2RoBERTaGPT-3CLIP Gemini Claude Instant 201920202021 2022 2023 2024 28 29
Model Architecture Key challenges in training and developing advanced AI models. Computtionl Resources Trends Demand for compute continues to grow, with model performance for AI tasks, they also require a different training requiring huge clusters of specialized accelera- programming model, tooling ecosystem, and set tors like GPUs and TPUs. However, the industry is un- of optimization techniques compared to tradition- dergoing a significant shift away from traditional CPUs al CPU-based workloads. Further, large models are towards these accelerator architectures optimized for usually trained across many accelerators and distribut- AI workloads. This transition brings significant chal- ed across many machines in parallel, requiring complex lenges in terms of infrastructure, tooling, and resource orchestration frameworks. management. New neural network designs and techniques are models like Falcon, Mixtral, and DBRX demonstrate To address these challenges, PyTorch introduced the enabling the development of larger, more capable the potential of these architectures, scoring high on The survey highlights the magnitude of this shift, with Fully Shared Data Parallel (FSDP). FSDP is a data models that can tackle increasingly complex tasks. performance benchmarks with significantly fewer pa- over 48% of respondents rating compute resource man- parallelism paradigm that shards model parameters, rameters and computational resources when compared agement as “most challenging” or “very challenging”. gradients, and optimizer states across data-parallel One new promising approach is the use of sparse to traditional models. Similarly, AI21 Labs’ Grok model workers, enabling more efficient memory usage and expert models, which allows for efficient training of showcases the power of sparse expert models in natural “CPUs consume about 80% of IT workloads today. GPUs training of larger models. massive networks by activating only relevant subsets language processing, excelling across a wide range of consume about 20%. That’s going to flip in the short term, of neurons for each input. This enables models to spe- language tasks while maintaining high efficiency. meaning 3 to 5 years. Many industry leaders that I’ve talked In addition to the challenge of compute resource cialize in different domains while still maintaining the to at Google and elsewhere believe that in 3 to 5 years, 80% management, model builders also face obstacles due ability to generalize across tasks. Recent open-source of IT workloads will be running on some type of architec- to a lack of suitable tools and frameworks. 38% of ture that is not CPU, but rather some type of chip architec- respondents indicated that the absence of AI-spe- ture like a GPU.” cific libraries, frameworks, and platforms is a major - Jon Barker, Customer Engineer, Google challenge holding back their AI projects. These tools are crucial for abstracting away the complexities of This rapid transition towards more costly GPU and distributed computing and accelerator programming, TPU-centric workloads presents a number of chal- allowing researchers to focus on model development lenges. While these accelerators offer unparalleled and experimentation. 30 31
Model Architecture Key challenges in training and developing advanced AI models. Computtionl Resources Trends Demand for compute continues to grow, with model performance for AI tasks, they also require a different training requiring huge clusters of specialized accelera- programming model, tooling ecosystem, and set tors like GPUs and TPUs. However, the industry is un- of optimization techniques compared to tradition- dergoing a significant shift away from traditional CPUs al CPU-based workloads. Further, large models are towards these accelerator architectures optimized for usually trained across many accelerators and distribut- AI workloads. This transition brings significant chal- ed across many machines in parallel, requiring complex lenges in terms of infrastructure, tooling, and resource orchestration frameworks. management. New neural network designs and techniques are models like Falcon, Mixtral, and DBRX demonstrate To address these challenges, PyTorch introduced the enabling the development of larger, more capable the potential of these architectures, scoring high on The survey highlights the magnitude of this shift, with Fully Shared Data Parallel (FSDP). FSDP is a data models that can tackle increasingly complex tasks.performance benchmarks with significantly fewer pa-over 48% of respondents rating compute resource man-parallelism paradigm that shards model parameters, rameters and computational resources when compared agement as “most challenging” or “very challenging”.gradients, and optimizer states across data-parallel One new promising approach is the use of sparse to traditional models. Similarly, AI21 Labs’ Grok model workers, enabling more efficient memory usage and expert models, which allows for efficient training of showcases the power of sparse expert models in natural “CPUs consume about 80% of IT workloads today. GPUs training of larger models. massive networks by activating only relevant subsets language processing, excelling across a wide range of consume about 20%. That’s going to flip in the short term, of neurons for each input. This enables models to spe-language tasks while maintaining high efficiency.meaning 3 to 5 years. Many industry leaders that I’ve talked In addition to the challenge of compute resource cialize in different domains while still maintaining the to at Google and elsewhere believe that in 3 to 5 years, 80% management, model builders also face obstacles due ability to generalize across tasks. Recent open-source of IT workloads will be running on some type of architec-to a lack of suitable tools and frameworks. 38% of ture that is not CPU, but rather some type of chip architec- respondents indicated that the absence of AI-spe- ture like a GPU.” cific libraries, frameworks, and platforms is a major - Jon Barker, Customer Engineer, Google challenge holding back their AI projects. These tools are crucial for abstracting away the complexities of This rapid transition towards more costly GPU and distributed computing and accelerator programming, TPU-centric workloads presents a number of chal- allowing researchers to focus on model development lenges. While these accelerators offer unparalleled and experimentation. 30 31
Unlocking AI Potential: Domin-Specific, Future Investments & Priorities Humn-Generted Dtsets Common approaches for data annotation. Top challenges in preparing high-quality training data for AI models. Data is the fuel that powers AI models, and the question answering, coding, and agentic use cases, ad- 69% of respondents rely on unstructured data like Managed labeling services allow companies to scale up quality, quantity, and diversity of that data is critical to vancements in AI capabilities will be bottlenecked by the text, images, audio, and video to train their models. labeling operations, reduce overhead, and access expert building effective, unbiased systems. The survey results supervision we can feed into these models. However, data quality emerges as the top challenge in annotators on-demand. Managed labeling services also highlight the importance of high-quality datasets, with acquiring training data, ranked as the largest obstacle handle project management, quality assurance, annotator labeling quality as the top challenge in preparing data Even if you train long enough with enough GPUs, you’ll by 35% of respondents. recruiting, and increasingly offer specialized expertise in for training models. Obtaining extremely high-quality get similar results with any modern model. It’s not about areas like coding, mathematics, and languages. labels while minimizing the time required to get that the model, it’s about the data that it was trained with. The To address this, 55% of organizations are leveraging labeled data is a significant hurdle for model builders. difference between performance is the volume and quality of internal labeling teams, while 50% engage specialized This highlights the need for efficient data labeling data, especially human feedback data. You absolutely need data labeling services and 29% leverage crowdsourcing. processes and tools that can maintain high standards it. That will determine your success. Organizations are scaling their annotation efforts with while expediting the labeling process. - Ashiqur Rahman, Machine Learning Researcher, managed labeling services, with 40% of users receiving Kimberly-Clark high-quality labeled data within one week to one month. Large, web-scraped datasets have been instrumental in pre-training foundation models. The next leap in Human-labeled data plays a critical role in aligning models capabilities will require more targeted, domain-specific with user preferences and real-world requirements. data that captures the nuances and edge cases that only Techniques like reinforcement learning from human human experts can provide.The advent of generative AI feedback (RLHF) can help guide models towards desired and large language models (LLMs) has fundamentally behaviors and outputs, but they require a steady stream of changed what it means to create high-quality training high-quality, human-generated labels and rankings. and evaluation data. For open-ended use cases, such as 32 33
Unlocking AI Potential: Domin-Specific, Future Investments & Priorities Humn-Generted Dtsets Common approaches for data annotation. Top challenges in preparing high-quality training data for AI models. Data is the fuel that powers AI models, and the question answering, coding, and agentic use cases, ad-69% of respondents rely on unstructured data like Managed labeling services allow companies to scale up quality, quantity, and diversity of that data is critical to vancements in AI capabilities will be bottlenecked by the text, images, audio, and video to train their models. labeling operations, reduce overhead, and access expert building effective, unbiased systems. The survey results supervision we can feed into these models.However, data quality emerges as the top challenge in annotators on-demand. Managed labeling services also highlight the importance of high-quality datasets, with acquiring training data, ranked as the largest obstacle handle project management, quality assurance, annotator labeling quality as the top challenge in preparing data Even if you train long enough with enough GPUs, you’ll by 35% of respondents.recruiting, and increasingly offer specialized expertise in for training models. Obtaining extremely high-quality get similar results with any modern model. It’s not about areas like coding, mathematics, and languages. labels while minimizing the time required to get that the model, it’s about the data that it was trained with. The To address this, 55% of organizations are leveraging labeled data is a significant hurdle for model builders. difference between performance is the volume and quality of internal labeling teams, while 50% engage specialized This highlights the need for efficient data labeling data, especially human feedback data. You absolutely need data labeling services and 29% leverage crowdsourcing. processes and tools that can maintain high standards it. That will determine your success. Organizations are scaling their annotation efforts with while expediting the labeling process.- Ashiqur Rahman, Machine Learning Researcher, managed labeling services, with 40% of users receiving Kimberly-Clarkhigh-quality labeled data within one week to one month. Large, web-scraped datasets have been instrumental in pre-training foundation models. The next leap in Human-labeled data plays a critical role in aligning models capabilities will require more targeted, domain-specific with user preferences and real-world requirements. data that captures the nuances and edge cases that only Techniques like reinforcement learning from human human experts can provide.The advent of generative AI feedback (RLHF) can help guide models towards desired and large language models (LLMs) has fundamentally behaviors and outputs, but they require a steady stream of changed what it means to create high-quality training high-quality, human-generated labels and rankings. and evaluation data. For open-ended use cases, such as 32 33
One new notable trend is the acquisition of proprietary By fusing diverse input modalities and investing in hu- data from platforms like Reddit, as exemplified by the man-in-the-loop pipelines, models can develop richer, recent multi-year data partnership between Reddit and more contextual representations that mirror how HUMAN Google. This deal, reportedly valued at $60 million humans process information and engage with their en- FEEDBACK per year, emphasizes the value placed on unique, hu- vironments. Organizations that can effectively harness man-generated content for training the next generation multimodal data and scale their labeling capabilities of models. will be well-positioned to unlock new frontiers in AI. However, simply acquiring vast amounts of data is not enough. To truly stay ahead of the curve, organizations must also invest in robust human-in-the-loop (HITL) DATA pipelines that can process and label data across an Data Flywheel ever-expanding range of modalities. As AI systems become more sophisticated, they will require not just text, but also speech, images, video, and even more complex data types like 3D scenes and sensor data. Moreover, the rise of reinforcement learning from human feedback (RLHF) has fundamentally changed MODEL TRAINING how models are evaluated. RLHF requires “on-policy” & OUTPUT human supervision, where human raters provide feedback on the actual outputs generated by the model during the training process. Additionally, traditional evaluation methods that rely on fixed sets of labels are no longer sufficient. Instead, organizations must conduct side-by-side comparisons of their old and new model responses across a large number of prompts before each release. This approach captures the nuances and edge cases that emerge as The demand for specific types of Scale’s Data Streams Going forward, we expect to see increased adoption models become more sophisticated and ensures that provides insights into the priorities and use cases of human-in-the-loop pipelines that leverage subject improvements are aligned with user expectations. driving AI development. Among the most sought-after matter experts to refine model outputs and provide Data Streams are: targeted feedback. This creates a virtuous “data Building scalable labeling programs that address mul- flywheel” effect, where model usage results in new timodal capabilities is a critical challenge for model 1. Coding, Reasoning, and Precise Instruction Following high-quality training data for continuous improvement. builders. It will require a combination of advanced tooling, specialized annotator training, and close 2. Languages Multimodal data collection spanning text, speech, collaboration between domain experts and machine images, and video will also be a key priority as organiza- learning teams. Managed labeling services with 3. Multimodal Data tions seek to build AI systems that can perceive, reason expertise across a wide range of modalities will be and interact more naturally. increasingly sought after to help organizations navigate this complex landscape. 34 35
One new notable trend is the acquisition of proprietary By fusing diverse input modalities and investing in hu- data from platforms like Reddit, as exemplified by the man-in-the-loop pipelines, models can develop richer, recent multi-year data partnership between Reddit and more contextual representations that mirror how HUMAN Google. This deal, reportedly valued at $60 million humans process information and engage with their en- FEEDBACK per year, emphasizes the value placed on unique, hu- vironments. Organizations that can effectively harness man-generated content for training the next generation multimodal data and scale their labeling capabilities of models. will be well-positioned to unlock new frontiers in AI. However, simply acquiring vast amounts of data is not enough. To truly stay ahead of the curve, organizations must also invest in robust human-in-the-loop (HITL) DATA pipelines that can process and label data across an Data Flywheelever-expanding range of modalities. As AI systems become more sophisticated, they will require not just text, but also speech, images, video, and even more complex data types like 3D scenes and sensor data. Moreover, the rise of reinforcement learning from human feedback (RLHF) has fundamentally changed MODEL TRAINING how models are evaluated. RLHF requires “on-policy” & OUTPUT human supervision, where human raters provide feedback on the actual outputs generated by the model during the training process. Additionally, traditional evaluation methods that rely on fixed sets of labels are no longer sufficient. Instead, organizations must conduct side-by-side comparisons of their old and new model responses across a large number of prompts before each release. This approach captures the nuances and edge cases that emerge as The demand for specific types of Scale’s Data Streams Going forward, we expect to see increased adoption models become more sophisticated and ensures that provides insights into the priorities and use cases of human-in-the-loop pipelines that leverage subject improvements are aligned with user expectations. driving AI development. Among the most sought-after matter experts to refine model outputs and provide Data Streams are:targeted feedback. This creates a virtuous “data Building scalable labeling programs that address mul- flywheel” effect, where model usage results in new timodal capabilities is a critical challenge for model 1. Coding, Reasoning, and Precise Instruction Followinghigh-quality training data for continuous improvement.builders. It will require a combination of advanced tooling, specialized annotator training, and close 2. LanguagesMultimodal data collection spanning text, speech, collaboration between domain experts and machine images, and video will also be a key priority as organiza-learning teams. Managed labeling services with 3. Multimodal Datations seek to build AI systems that can perceive, reason expertise across a wide range of modalities will be and interact more naturally.increasingly sought after to help organizations navigate this complex landscape. 34 35
Evluting Model Performnce Evlute AI Evaluation critera for models in use 68% Reliability 67% Performance 62% Security 54% Safety 6% N/A As foundation models grow in Despite this focus on evalua- ganizations are moving towards capability and impact, compre- tion, developing robust evalua- comprehensive private test suites hensive model evaluation has tion frameworks is an evolving that probe model behavior across become paramount whether you challenge. Models must be assessed diverse domains and capabilities. are building or applying models. holistically, accounting for perfor- Universally agreed upon 3rd party In contrast to common headlines, mance on real-world use cases as benchmarks are crucial for objec- assessing foundation models is not well as potential risks. Traditional tively evaluating and comparing just about safety. In fact, perfor- academic benchmarks are generally the performance of large language mance, reliability, and security were not representative of production models. Researchers, develop- indicated as the top three reasons scenarios, and models have been ers, and users can select models survey respondents evaluate overfitted to these existing bench- based on standardized transparent models - with safety ranking as a marks due to their presence in metrics. lower priority. the public domain. Leading or- 36 37
Evluting Model Performnce Evlute AIEvaluation critera for models in use 68% Reliability 67% Performance 62% Security 54% Safety 6% N/A As foundation models grow in Despite this focus on evalua- ganizations are moving towards capability and impact, compre- tion, developing robust evalua- comprehensive private test suites hensive model evaluation has tion frameworks is an evolving that probe model behavior across become paramount whether you challenge. Models must be assessed diverse domains and capabilities. are building or applying models. holistically, accounting for perfor- Universally agreed upon 3rd party In contrast to common headlines, mance on real-world use cases as benchmarks are crucial for objec- assessing foundation models is not well as potential risks. Traditional tively evaluating and comparing just about safety. In fact, perfor- academic benchmarks are generally the performance of large language mance, reliability, and security were not representative of production models. Researchers, develop- indicated as the top three reasons scenarios, and models have been ers, and users can select models survey respondents evaluate overfitted to these existing bench- based on standardized transparent models - with safety ranking as a marks due to their presence in metrics. lower priority. the public domain. Leading or- 36 37
Evaluation practices for model performance. To understand current evaluation practices, the survey asked respondents how they measure model performance. The top approaches are illustrated in the figure, left. The data shows that automated model metrics and human preference ranking are the fastest ways to identify issues, with over 70% of respondents discovering problems within one week. This high- lights the value of quantitative and qual- itative evaluation approaches to rapidly surface model performance problems. The prevalence of human evaluations is notable (41%), reflecting the importance of subjective judgments in assessing generative outputs. Techniques like preference ranking, where human raters compare model samples, can capture nuanced quality distinctions. The survey results suggest that a multi-faceted evaluation strategy is necessary, as no single approach dominates. While automated metrics and Model builders who apply AI business impact assessments are widely indicated that they evaluate used, the data indicates the need to models or applications. 87% incorporate a variety of quantitative and qualitative techniques to comprehensive- ly evaluate models. Enterprises who apply AI indicated that they evaluate 72% models or applications. When asked why they conduct model evaluations, 69% of respondents selected performance, another 69% selected reli- ability and 63% selected security as main objectives. Stress testing models is an important defense against failure modes such as hallucination and bias. 38 39
Evaluation practices for model performance. To understand current evaluation practices, the survey asked respondents how they measure model performance. The top approaches are illustrated in the figure, left. The data shows that automated model metrics and human preference ranking are the fastest ways to identify issues, with over 70% of respondents discovering problems within one week. This high- lights the value of quantitative and qual- itative evaluation approaches to rapidly surface model performance problems. The prevalence of human evaluations is notable (41%), reflecting the importance of subjective judgments in assessing generative outputs. Techniques like preference ranking, where human raters compare model samples, can capture nuanced quality distinctions. The survey results suggest that a multi-faceted evaluation strategy is necessary, as no single approach dominates. While automated metrics and Model builders who apply AI business impact assessments are widely indicated that they evaluate used, the data indicates the need to models or applications. 87% incorporate a variety of quantitative and qualitative techniques to comprehensive- ly evaluate models. Enterprises who apply AI indicated that they evaluate 72% models or applications. When asked why they conduct model evaluations, 69% of respondents selected performance, another 69% selected reli- ability and 63% selected security as main objectives. Stress testing models is an important defense against failure modes such as hallucination and bias. 38 39
Techniques like red teaming, where expert testers try to elicit unsafe behaviors, can surface vulnera- bilities. Careful prompt engineering can also help assess models’ resilience against malicious prompts or out-of-distribution inputs. The results highlight the importance of continuous monitoring, as models can degrade or exhibit new issues over time. Over 40% of respondents evaluate their models following any changes or prior to major releases, highlighting the shift towards a continuous evaluation that goes beyond one-time assessments. While model evaluation plays a crucial role in measuring AI performance, leaders responsible for applying AI in their organizations must also demon- strate tangible business outcomes. Almost half of respondents evaluate models based on their direct impact on KPIs like operational efficiency or customer satisfaction. Grounding evaluations in downstream outcomes ensures that models are not just technically proficient but actually valuable in practice. 40 41
Techniques like red teaming, where expert testers try to elicit unsafe behaviors, can surface vulnera- bilities. Careful prompt engineering can also help assess models’ resilience against malicious prompts or out-of-distribution inputs. The results highlight the importance of continuous monitoring, as models can degrade or exhibit new issues over time. Over 40% of respondents evaluate their models following any changes or prior to major releases, highlighting the shift towards a continuous evaluation that goes beyond one-time assessments. While model evaluation plays a crucial role in measuring AI performance, leaders responsible for applying AI in their organizations must also demon- strate tangible business outcomes. Almost half of respondents evaluate models based on their direct impact on KPIs like operational efficiency or customer satisfaction. Grounding evaluations in downstream outcomes ensures that models are not just technically proficient but actually valuable in practice. 40 41
Model evaluation challenges: gaps in benchmarking for model builders and enterprises applying AI “Evaluating generative AI performance is complex due to evolving benchmarks, data drift, model versioning, and the need to coordinate across diverse teams. The key question is how the model performs on specific data and use cases... Centralized oversight of the data flow is essential for effective model evaluation and risk management in order to achieve high acceptance rates from developers and other stakeholders.” Babar Bhai, IBM, AI CUSTOMER SUCCESS LEAD Chllenges with model evlution tody Despite progress, many gaps remain in current model The data reveals room for improvement in measuring evaluation practices. the business impact of AI models. For key outcomes like revenue, profitability, and strategic decision-mak- Performance and usability benchmarks are critical to ing, only half of the organizations are assessing ensure models meet rising user expectations while business impact. This represents an opportunity for vertical-specific standards will be key as AI permeates enterprises to more clearly link model performance to different sectors. Industry groups like the National tangible business results, ensuring that AI investments Institute of Standards and Technology (NIST) are are delivering real value. working to define comprehensive evaluation standards. Scale’s Safety, Evaluations, and Analysis Lab (SEAL) is also working to develop robust evaluation frameworks. 42 43
Model evaluation challenges: gaps in benchmarking for model builders and enterprises applying AI “Evaluating generative AI performance is complex due to evolving benchmarks, data drift, model versioning, and the need to coordinate across diverse teams. The key question is how the model performs on specific data and use cases... Centralized oversight of the data flow is essential for effective model evaluation and risk management in order to achieve high acceptance rates from developers and other stakeholders.” Babar Bhai, IBM, AI CUSTOMER SUCCESS LEADChllenges with model evlution tody Despite progress, many gaps remain in current model The data reveals room for improvement in measuring evaluation practices. the business impact of AI models. For key outcomes like revenue, profitability, and strategic decision-mak- Performance and usability benchmarks are critical to ing, only half of the organizations are assessing ensure models meet rising user expectations while business impact. This represents an opportunity for vertical-specific standards will be key as AI permeates enterprises to more clearly link model performance to different sectors. Industry groups like the National tangible business results, ensuring that AI investments Institute of Standards and Technology (NIST) are are delivering real value. working to define comprehensive evaluation standards. Scale’s Safety, Evaluations, and Analysis Lab (SEAL) is also working to develop robust evaluation frameworks. 42 43
Practices for evaluating AI systems in production “As AI systems become more advanced and influential, it’s crucial that we prioritize AI safety. The rapid progress in large language models and generative AI is both awe-in- spiring and sobering - while these technologies could help solve some of humanity’s greatest challenges, they also pose catastrophic risks if developed without sufficient safeguards. At the Center for AI Safety, our research focuses on the important problem of AI safety: mitigating the various risks posed by AI systems. Evluting AI Systems in Production We also need proactive governance strategies to navigate Robust evaluation practices are essential not just external evaluation platforms, 49% use proprietary the high-stakes landscape of powerful AI, including estab- during model development, but also when deploying internal platforms, 38% adopt third-party platforms and lishing international cooperation, safety standards, and and monitoring AI systems in real-world production 21% engage external consultants. environments. regulatory oversight. While the era of advanced AI presents These results underscore the complexity of validating The survey highlights how both model builders and en- AI system performance, safety, and alignment with re- tremendous potential, we must not underestimate the risks terprises are investing in evaluation capabilities. On the al-world operating conditions and business objectives. and challenges ahead. It’s crucial that the AI community “Build” side, organizations recognize the importance of Effective evaluation requires a blend of skilled in-house comprehensive evaluations and employ a combination teams, robust tools and frameworks, and external spe- comes together to prioritize safety, so we can chart a course of internal dashboards and external platforms to gain cialist support. towards a future where AI is a profound positive force for a holistic understanding of model performance. 46% of organizations have internal teams with dedicated Looking ahead, evaluation methodology must evolve in the world.” test and evaluation platforms, while 64% leverage lockstep with AI capabilities. Multidisciplinary research internal proprietary platforms. Adoption of third-party at the intersection of machine learning, software engi- evaluation consultancies (23%) and platforms (40%) neering, and social science is needed to define rigorous Dan Hendrycks, is also prevalent, demonstrating the value of external standards. Scalable infrastructure for human-in-the- expertise and tools in the evaluation process. loop evaluation pipelines will also be critical. With CENTER FOR AI SAFETY (CAIS) sustained effort and investment, the industry can build For enterprises focused on “Applying” AI, the invest- generative models that are not only powerful but truly ment patterns are similar but with a blend of internal reliable and beneficial. and external solutions. 42% have internal teams using 44 45
Practices for evaluating AI systems in production “As AI systems become more advanced and influential, it’s crucial that we prioritize AI safety. The rapid progress in large language models and generative AI is both awe-in- spiring and sobering - while these technologies could help solve some of humanity’s greatest challenges, they also pose catastrophic risks if developed without sufficient safeguards. At the Center for AI Safety, our research focuses on the important problem of AI safety: mitigating the various risks posed by AI systems. Evluting AI Systems in Production We also need proactive governance strategies to navigate Robust evaluation practices are essential not just external evaluation platforms, 49% use proprietary the high-stakes landscape of powerful AI, including estab- during model development, but also when deploying internal platforms, 38% adopt third-party platforms and lishing international cooperation, safety standards, and and monitoring AI systems in real-world production 21% engage external consultants. environments. regulatory oversight. While the era of advanced AI presents These results underscore the complexity of validating The survey highlights how both model builders and en-AI system performance, safety, and alignment with re-tremendous potential, we must not underestimate the risks terprises are investing in evaluation capabilities. On the al-world operating conditions and business objectives. and challenges ahead. It’s crucial that the AI community “Build” side, organizations recognize the importance of Effective evaluation requires a blend of skilled in-house comprehensive evaluations and employ a combination teams, robust tools and frameworks, and external spe-comes together to prioritize safety, so we can chart a course of internal dashboards and external platforms to gain cialist support.towards a future where AI is a profound positive force for a holistic understanding of model performance. 46% of organizations have internal teams with dedicated Looking ahead, evaluation methodology must evolve in the world.” test and evaluation platforms, while 64% leverage lockstep with AI capabilities. Multidisciplinary research internal proprietary platforms. Adoption of third-party at the intersection of machine learning, software engi- evaluation consultancies (23%) and platforms (40%) neering, and social science is needed to define rigorous Dan Hendrycks, is also prevalent, demonstrating the value of external standards. Scalable infrastructure for human-in-the- expertise and tools in the evaluation process.loop evaluation pipelines will also be critical. With CENTER FOR AI SAFETY (CAIS) sustained effort and investment, the industry can build For enterprises focused on “Applying” AI, the invest-generative models that are not only powerful but truly ment patterns are similar but with a blend of internal reliable and beneficial. and external solutions. 42% have internal teams using 44 45
Conclusion About Scle Methodology Scale is fueling the generative AI revolu- This survey was conducted online within the United tion. Built on a foundation of high-quality States by Scale AI from February 20, 2024, to March 29, Whether you are building or applying AI, data and expert insight, Scale powers the 2024. We received 2,302 responses from ML prac- world’s most advanced models. Our years titioners (e.g., ML engineers, data scientists, devel- of deep partnership with every major opment operations, etc.) and leaders involved with model optimization and evaluation is key model builder enables our platform to AI in their companies. Participants who reported no empower any organization to apply and involvement in AI or ML projects were excluded from to unlock performance and ROI. evaluate AI. the dataset, resulting in a final sample size of 1800 respondents. scale.com The pace of innovation for generative AI continues A quarter of the respondents identified themselves as to accelerate. While the 2023 AI Readiness Report belonging to the Software and Internet/Telecommu- focused on how enterprises could adopt AI, this year’s nications industry (28%), with the Financial Services/ report examined challenges and best practices to Insurance Industry following closely behind at 15%. apply, build, and evaluate AI. The two most significant Business Services accounted for 7%, while the Gov- trends to emerge in our analysis are: ernment and Defense Industry represented 4% of the respondents. Among these industries, a majority of 1. The growing need for model eval- respondents specified their employment within the uation frameworks and private Information Technology department (33%). benchmarks. In terms of seniority within their organizations, nearly 2. The continued challenges a quarter of respondents (24%) identified themselves of optimizing models for as Team Leads, 22% as department heads, and 5% as specific use cases without owners. Sixty-six percent (66%) of respondents report sufficient tooling for involvement in AI model application and customization data preparation, model (applying AI), while 34% are directly engaged in de- training, and deploy- veloping foundational generative AI models (building ment. AI). Consequently, a significant portion of respondents (46%) represent organizations at an advanced stage of At Scale, our mission is to AI/ML adoption, with one to multiple models deployed accelerate the develop- to production and undergoing regular retraining. ment of AI applications. Approximately 26% are in the process of The Scale Zeitgeist: AI developing their inaugural model, while Readiness Report supports 23% are in the phase of evaluating that mission. We will potential use cases, underscoring continue to shed light on the significance and enthu- the latest trends, challenges, siasm for AI/ML project and what it really takes to development. build, apply, and evaluate AI. 46 47
Conclusion About Scle Methodology Scale is fueling the generative AI revolu- This survey was conducted online within the United tion. Built on a foundation of high-quality States by Scale AI from February 20, 2024, to March 29, Whether you are building or applying AI, data and expert insight, Scale powers the 2024. We received 2,302 responses from ML prac- world’s most advanced models. Our years titioners (e.g., ML engineers, data scientists, devel- of deep partnership with every major opment operations, etc.) and leaders involved with model optimization and evaluation is key model builder enables our platform to AI in their companies. Participants who reported no empower any organization to apply and involvement in AI or ML projects were excluded from to unlock performance and ROI.evaluate AI. the dataset, resulting in a final sample size of 1800 respondents. scale.com The pace of innovation for generative AI continues A quarter of the respondents identified themselves as to accelerate. While the 2023 AI Readiness Report belonging to the Software and Internet/Telecommu- focused on how enterprises could adopt AI, this year’s nications industry (28%), with the Financial Services/ report examined challenges and best practices to Insurance Industry following closely behind at 15%. apply, build, and evaluate AI. The two most significant Business Services accounted for 7%, while the Gov- trends to emerge in our analysis are: ernment and Defense Industry represented 4% of the respondents. Among these industries, a majority of 1. The growing need for model eval- respondents specified their employment within the uation frameworks and private Information Technology department (33%). benchmarks. In terms of seniority within their organizations, nearly 2. The continued challenges a quarter of respondents (24%) identified themselves of optimizing models for as Team Leads, 22% as department heads, and 5% as specific use cases without owners. Sixty-six percent (66%) of respondents report sufficient tooling for involvement in AI model application and customization data preparation, model (applying AI), while 34% are directly engaged in de- training, and deploy- veloping foundational generative AI models (building ment. AI). Consequently, a significant portion of respondents (46%) represent organizations at an advanced stage of At Scale, our mission is to AI/ML adoption, with one to multiple models deployed accelerate the develop- to production and undergoing regular retraining. ment of AI applications. Approximately 26% are in the process of The Scale Zeitgeist: AI developing their inaugural model, while Readiness Report supports 23% are in the phase of evaluating that mission. We will potential use cases, underscoring continue to shed light on the significance and enthu- the latest trends, challenges, siasm for AI/ML project and what it really takes to development. build, apply, and evaluate AI. 46 47