Unlocking AI Potential: Domin-Specific, Future Investments & Priorities Humn-Generted Dtsets Common approaches for data annotation. Top challenges in preparing high-quality training data for AI models. Data is the fuel that powers AI models, and the question answering, coding, and agentic use cases, ad- 69% of respondents rely on unstructured data like Managed labeling services allow companies to scale up quality, quantity, and diversity of that data is critical to vancements in AI capabilities will be bottlenecked by the text, images, audio, and video to train their models. labeling operations, reduce overhead, and access expert building effective, unbiased systems. The survey results supervision we can feed into these models. However, data quality emerges as the top challenge in annotators on-demand. Managed labeling services also highlight the importance of high-quality datasets, with acquiring training data, ranked as the largest obstacle handle project management, quality assurance, annotator labeling quality as the top challenge in preparing data Even if you train long enough with enough GPUs, you’ll by 35% of respondents. recruiting, and increasingly offer specialized expertise in for training models. Obtaining extremely high-quality get similar results with any modern model. It’s not about areas like coding, mathematics, and languages. labels while minimizing the time required to get that the model, it’s about the data that it was trained with. The To address this, 55% of organizations are leveraging labeled data is a significant hurdle for model builders. difference between performance is the volume and quality of internal labeling teams, while 50% engage specialized This highlights the need for efficient data labeling data, especially human feedback data. You absolutely need data labeling services and 29% leverage crowdsourcing. processes and tools that can maintain high standards it. That will determine your success. Organizations are scaling their annotation efforts with while expediting the labeling process. - Ashiqur Rahman, Machine Learning Researcher, managed labeling services, with 40% of users receiving Kimberly-Clark high-quality labeled data within one week to one month. Large, web-scraped datasets have been instrumental in pre-training foundation models. The next leap in Human-labeled data plays a critical role in aligning models capabilities will require more targeted, domain-specific with user preferences and real-world requirements. data that captures the nuances and edge cases that only Techniques like reinforcement learning from human human experts can provide.The advent of generative AI feedback (RLHF) can help guide models towards desired and large language models (LLMs) has fundamentally behaviors and outputs, but they require a steady stream of changed what it means to create high-quality training high-quality, human-generated labels and rankings. and evaluation data. For open-ended use cases, such as 32 33
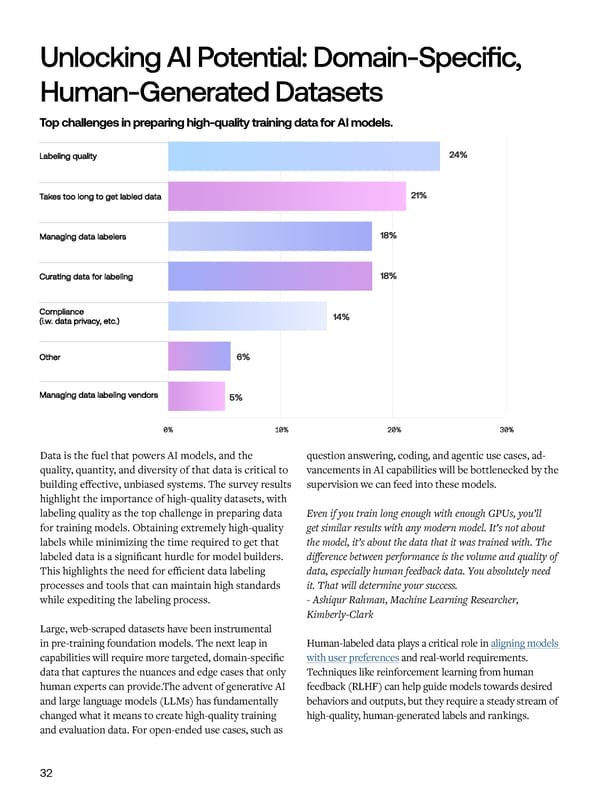