Model evaluation challenges: gaps in benchmarking for model builders and enterprises applying AI “Evaluating generative AI performance is complex due to evolving benchmarks, data drift, model versioning, and the need to coordinate across diverse teams. The key question is how the model performs on specific data and use cases... Centralized oversight of the data flow is essential for effective model evaluation and risk management in order to achieve high acceptance rates from developers and other stakeholders.” Babar Bhai, IBM, AI CUSTOMER SUCCESS LEAD Chllenges with model evlution tody Despite progress, many gaps remain in current model The data reveals room for improvement in measuring evaluation practices. the business impact of AI models. For key outcomes like revenue, profitability, and strategic decision-mak- Performance and usability benchmarks are critical to ing, only half of the organizations are assessing ensure models meet rising user expectations while business impact. This represents an opportunity for vertical-specific standards will be key as AI permeates enterprises to more clearly link model performance to different sectors. Industry groups like the National tangible business results, ensuring that AI investments Institute of Standards and Technology (NIST) are are delivering real value. working to define comprehensive evaluation standards. Scale’s Safety, Evaluations, and Analysis Lab (SEAL) is also working to develop robust evaluation frameworks. 42 43
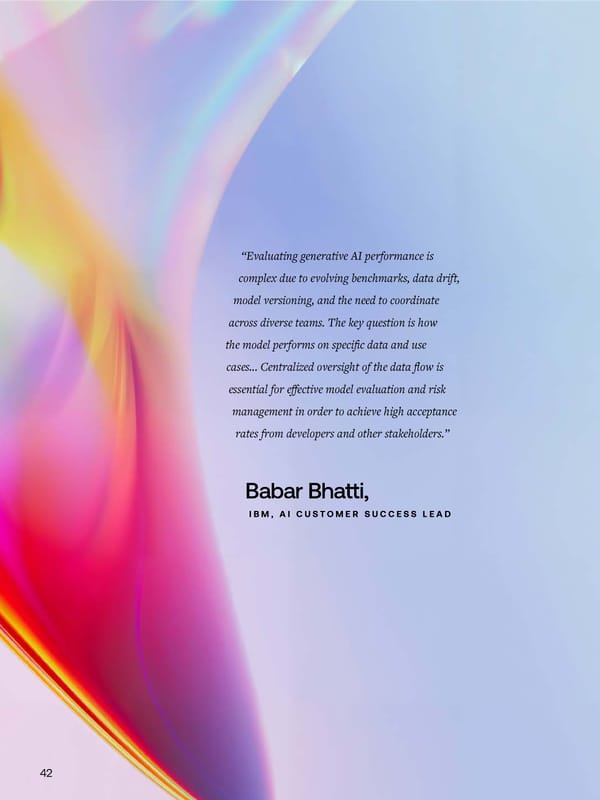