Practices for evaluating AI systems in production “As AI systems become more advanced and influential, it’s crucial that we prioritize AI safety. The rapid progress in large language models and generative AI is both awe-in- spiring and sobering - while these technologies could help solve some of humanity’s greatest challenges, they also pose catastrophic risks if developed without sufficient safeguards. At the Center for AI Safety, our research focuses on the important problem of AI safety: mitigating the various risks posed by AI systems. Evluting AI Systems in Production We also need proactive governance strategies to navigate Robust evaluation practices are essential not just external evaluation platforms, 49% use proprietary the high-stakes landscape of powerful AI, including estab- during model development, but also when deploying internal platforms, 38% adopt third-party platforms and lishing international cooperation, safety standards, and and monitoring AI systems in real-world production 21% engage external consultants. environments. regulatory oversight. While the era of advanced AI presents These results underscore the complexity of validating The survey highlights how both model builders and en-AI system performance, safety, and alignment with re-tremendous potential, we must not underestimate the risks terprises are investing in evaluation capabilities. On the al-world operating conditions and business objectives. and challenges ahead. It’s crucial that the AI community “Build” side, organizations recognize the importance of Effective evaluation requires a blend of skilled in-house comprehensive evaluations and employ a combination teams, robust tools and frameworks, and external spe-comes together to prioritize safety, so we can chart a course of internal dashboards and external platforms to gain cialist support.towards a future where AI is a profound positive force for a holistic understanding of model performance. 46% of organizations have internal teams with dedicated Looking ahead, evaluation methodology must evolve in the world.” test and evaluation platforms, while 64% leverage lockstep with AI capabilities. Multidisciplinary research internal proprietary platforms. Adoption of third-party at the intersection of machine learning, software engi- evaluation consultancies (23%) and platforms (40%) neering, and social science is needed to define rigorous Dan Hendrycks, is also prevalent, demonstrating the value of external standards. Scalable infrastructure for human-in-the- expertise and tools in the evaluation process.loop evaluation pipelines will also be critical. With CENTER FOR AI SAFETY (CAIS) sustained effort and investment, the industry can build For enterprises focused on “Applying” AI, the invest-generative models that are not only powerful but truly ment patterns are similar but with a blend of internal reliable and beneficial. and external solutions. 42% have internal teams using 44 45
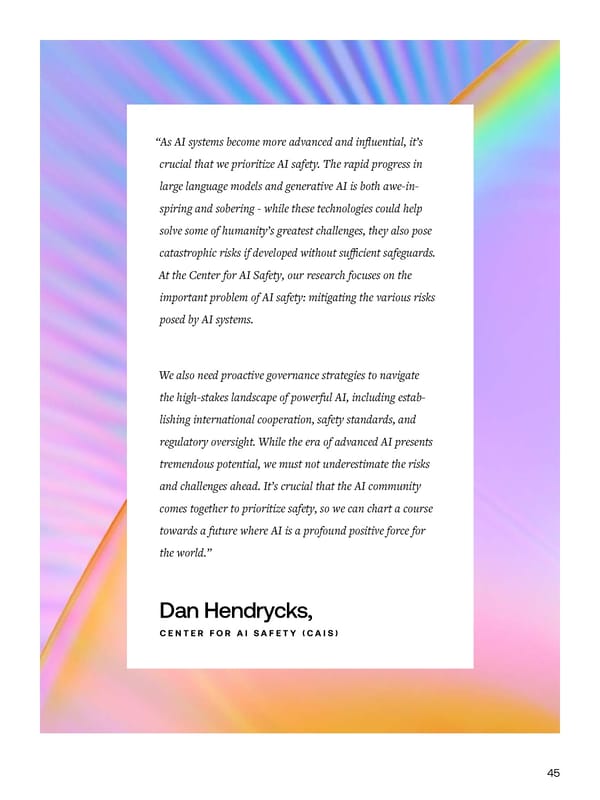