One new notable trend is the acquisition of proprietary By fusing diverse input modalities and investing in hu- data from platforms like Reddit, as exemplified by the man-in-the-loop pipelines, models can develop richer, recent multi-year data partnership between Reddit and more contextual representations that mirror how HUMAN Google. This deal, reportedly valued at $60 million humans process information and engage with their en- FEEDBACK per year, emphasizes the value placed on unique, hu- vironments. Organizations that can effectively harness man-generated content for training the next generation multimodal data and scale their labeling capabilities of models. will be well-positioned to unlock new frontiers in AI. However, simply acquiring vast amounts of data is not enough. To truly stay ahead of the curve, organizations must also invest in robust human-in-the-loop (HITL) DATA pipelines that can process and label data across an Data Flywheelever-expanding range of modalities. As AI systems become more sophisticated, they will require not just text, but also speech, images, video, and even more complex data types like 3D scenes and sensor data. Moreover, the rise of reinforcement learning from human feedback (RLHF) has fundamentally changed MODEL TRAINING how models are evaluated. RLHF requires “on-policy” & OUTPUT human supervision, where human raters provide feedback on the actual outputs generated by the model during the training process. Additionally, traditional evaluation methods that rely on fixed sets of labels are no longer sufficient. Instead, organizations must conduct side-by-side comparisons of their old and new model responses across a large number of prompts before each release. This approach captures the nuances and edge cases that emerge as The demand for specific types of Scale’s Data Streams Going forward, we expect to see increased adoption models become more sophisticated and ensures that provides insights into the priorities and use cases of human-in-the-loop pipelines that leverage subject improvements are aligned with user expectations. driving AI development. Among the most sought-after matter experts to refine model outputs and provide Data Streams are:targeted feedback. This creates a virtuous “data Building scalable labeling programs that address mul- flywheel” effect, where model usage results in new timodal capabilities is a critical challenge for model 1. Coding, Reasoning, and Precise Instruction Followinghigh-quality training data for continuous improvement.builders. It will require a combination of advanced tooling, specialized annotator training, and close 2. LanguagesMultimodal data collection spanning text, speech, collaboration between domain experts and machine images, and video will also be a key priority as organiza-learning teams. Managed labeling services with 3. Multimodal Datations seek to build AI systems that can perceive, reason expertise across a wide range of modalities will be and interact more naturally.increasingly sought after to help organizations navigate this complex landscape. 34 35
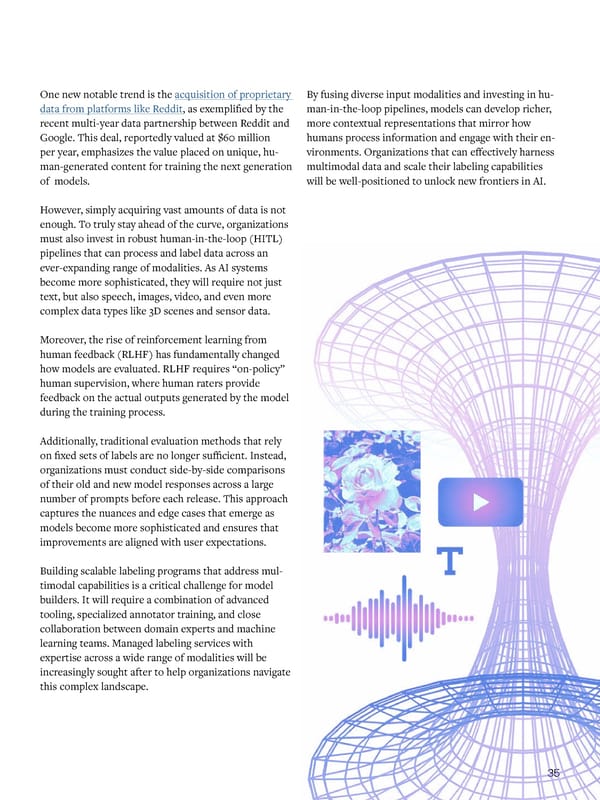