Evaluation practices for model performance. To understand current evaluation practices, the survey asked respondents how they measure model performance. The top approaches are illustrated in the figure, left. The data shows that automated model metrics and human preference ranking are the fastest ways to identify issues, with over 70% of respondents discovering problems within one week. This high- lights the value of quantitative and qual- itative evaluation approaches to rapidly surface model performance problems. The prevalence of human evaluations is notable (41%), reflecting the importance of subjective judgments in assessing generative outputs. Techniques like preference ranking, where human raters compare model samples, can capture nuanced quality distinctions. The survey results suggest that a multi-faceted evaluation strategy is necessary, as no single approach dominates. While automated metrics and Model builders who apply AI business impact assessments are widely indicated that they evaluate used, the data indicates the need to models or applications. 87% incorporate a variety of quantitative and qualitative techniques to comprehensive- ly evaluate models. Enterprises who apply AI indicated that they evaluate 72% models or applications. When asked why they conduct model evaluations, 69% of respondents selected performance, another 69% selected reli- ability and 63% selected security as main objectives. Stress testing models is an important defense against failure modes such as hallucination and bias. 38 39
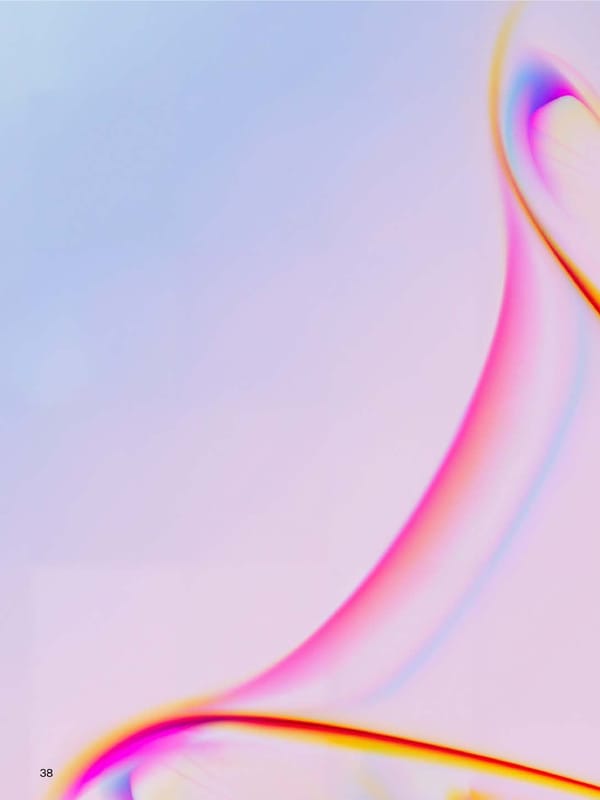