“RAG aims to address a key challenge with LLMs - while they are very creative, they lack factual understanding of the world and struggle to explain their reasoning. RAG tackles this by connecting LLMs to known data sources, like a bank’s general ledger, using vector search on a database. This augments the LLM prompts with relevant facts. However, implementing RAG presents its own challenges. It requires creating and maintaining the external data connection, setting up a fast vector database, and designing vector representations of the data for efficient search. Companies need to consider if they require a purpose-built database optimized for vector search. Keeping this vectorized representation of truth up-to-date is tricky. As the underlying data sources change over time and users ask new questions, the vector database needs to evolve as well. Deciding if and how to incorporate user assumptions into the vector representations is a philosophical question that also has practical implications for implementation. The industry is still grappling with how to design RAG systems that can continually improve over time.” Jon Barker, CUSTOMER ENGINEER, GOOGLE 24 25
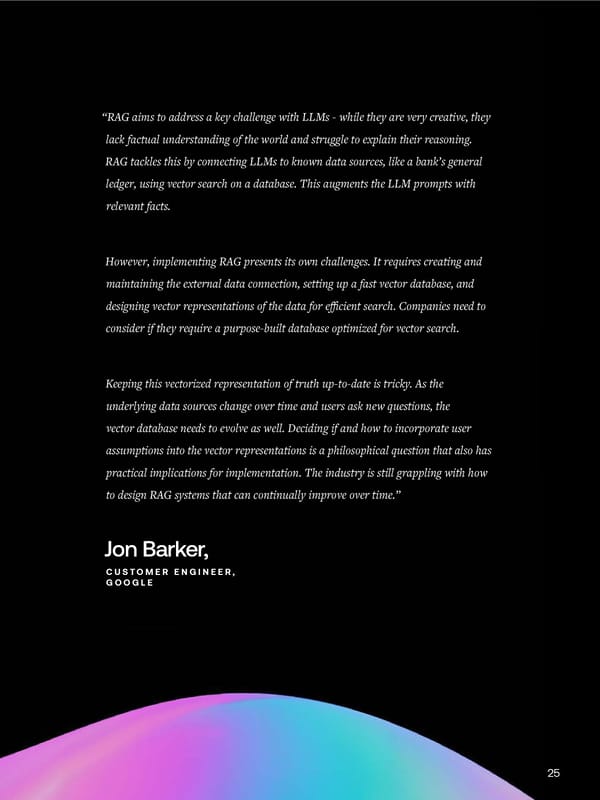